More is different.
God is in the details.
NEWS
- Dates
- March 25-29, 2024
- Conference
- SQAI-NCTS Workshop on Tensor Network and Quantum Embedding (Hongo Campus, The University of Tokyo)
- Title
- Optimizing the structure of tree tensor network for quantum generative modeling using mutual information-based approach
- Abstract
- Generative modeling is a crucial task in the field of machine learning. Recently, there have been several proposals for generative models on quantum devices. We can efficiently optimize generative models defined by tensor network states, but their performance largely depends on the geometrical structure of the tensor network. To tackle this issue, we have proposed an optimization method for the network structure in the tree tensor network class, based on the least mutual information principle. Generative modeling with an optimized network structure has better performance than a fixed network structure. Moreover, by embedding data dependencies into the tree structure based on the least mutual information principle, we can geometrically represent the correlations in the data.
- Book title
- Advanced Mathematical Science for Mobility Society
- Editors
- Kazushi Ikeda, Yoshiumi Kawamura, Kazuhisa Makino, Satoshi Tsujimoto, Nobuo Yamashita, Shintaro Yoshizawa, Hanna Sumita
- Publisher
- Springer Singapore
- Reference
- ISBN 978-981-99-9771-8 ISBN 978-981-99-9772-5 (eBook)
- Title
- Chapter 5 "Application of Tensor Network Formalism for Processing Tensor Data"
- Authors
- Kenji Harada, Hiroaki Matsueda, and Tsuyoshi Okubo
- Web page
- Open access
- Date
- Jan 26, 2024
- Conference
- 2024 Annual Meeting of the Physical Society of Taiwan, Topical Symposia:Many-body systems and advanced numerical methods
- Title
- Optimizing tensor network structure
- Date
- Jan 22, 2024
- Conference
- Mini-workshop: Tensor Network algorithms and applications 2024 (Taipei, Taiwan)
- Title
- Optimizing tensor network structure
- Date
- Aug 22, 2023
- Conference
- Tensor Network States: Algorithms and Applications 2023 (Shanghai, China)
- Title
- Tensor network study of one-dimensional stochastic processes
- Date
- Aug 8, 2023
- Conference
- The 28th International Conference on Statistical Physics, Statphys28 (Tokyot, Japan)
- Title
- Renormalization of non-equilibrium critical points in one-dimensional stochastic processes by tensor networks
- Abstract
-
Non-equilibrium critical points often hold scaling invariance in the spatial and temporal directions. As seen in equilibrium cases, we know various universality classes of non-equilibrium critical points in stochastic processes. However, the direct renormalization of statistical processes is technically difficult. Recently, renormalization using tensor network representation was proposed and extended[1-4], and it is quite successful in equilibrium critical points. We extend the approach to stochastic processes using oblique projectors in the tensor renormalization group with higher-order singular value decomposition[5]. We report the universal property of time-evolution operators of one-dimensional contact processes of which critical points belong to the (1+1)-dimensional directed percolation(DP) universality class. The renormalized time-evolution operator has a universal spectrum structure of the (1+1)-dimensional DP universality class in spatial and temporal directions.
[1] M. Levin and C. P. Nave, Tensor Renormalization Group Approach to Two-Dimensional Classical Lattice Models, Physical Review Letters 99, 120601 (2007).
[2] Z. Y. Xie, J. Chen, M. P. Qin, J. W. Zhu, Y. P. L., and T. Xiang, Coarse-Graining Renormalization by Higher-Order Singular Value Decomposition, Physical Review B 86, 045139 (2012).
[3] G. Evenbly and G. Vidal, Tensor Network Renormalization, Physical Review Letters 115, 180405 (2015).
[4] K. Harada, Entanglement Branching Operator, Physical Review B 97, 045124 (2018).
[5] K. Harada, Universal spectrum structure at nonequilibrium critical points in the (1+1)-dimensional directed percolation, arXiv:2008.10807.
TOPICS
Toolkit of Bayesian Scaling Analysis
Reference application software of a new scaling analysis method of critical phenomena based on Bayesian inference.
To demo To detailsMonte Carlo simulations
This demonstration shows a Monte Carlo simulation of the two-dimensional Ising model by three algorithms: Metropolis, Swendsen-Wang, and Wolff algorithms.
To demoABOUT
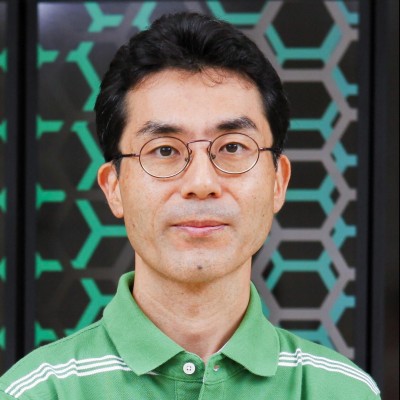
Kenji Harada
(
原田健自
)
Assistant Professor,
Graduate School of Informatics, Kyoto University, Japan.
harada.kenji.8e@kyoto-u.ac.jp
Room 203, Research Bldg. No.8, Yoshida Campus, Kyoto Univ., Kyoto, 606-8501, Japan.
Map (No.59)